Exploring the potential of machine learning in quantum technology
Ying-Cheng Lai, a Regents’ Professor at Arizona State University’s School of Electrical, Computer, and Energy Engineering, has spent 30 years studying complex quantum systems and unpredictable behavior in physics. Now he’s taking on a big challenge: controlling a mysterious quantum phenomenon called entanglement.
Entanglement is a fascinating behavior where particles, such as electrons or photons, become interconnected no matter how far apart they are – almost like siblings who share the same thoughts when they aren’t together. In physics, this connection is crucial for building super-powerful quantum computers and ultra-secure communication systems, but it’s incredibly tricky to control.
That’s where Lai’s research comes in.
Lai’s team is developing a new way to control entanglement using machine learning, a type of artificial intelligence that learns and improves from experience. Their goal is to create and stabilize entanglement using light and tiny mechanical parts, known as quantum optomechanical systems. These systems can produce precise quantum measurements and boost computational power, making them valuable for both fundamental physics research and practical applications.
Traditional methods of controlling quantum systems rely on detailed mathematical models, but these models aren’t always available or accurate. Lai’s approach is different. Instead of using a model, his machine learning algorithm learns by interacting with the system and adjusting its actions based on the results – adapting and learning in real-time.
One major challenge with quantum optomechanical systems is their sensitivity to their environment. Small disturbances can easily disrupt their function. To overcome this, Lai’s team developed a two-phase training process for their machine learning algorithm. In the first phase, the algorithm learns from simpler, more predictable situations. In the second phase, it applies this knowledge to more complex and realistic scenarios.
So far, they’ve shown that their machine learning-based control could potentially achieve and maintain any desired level of entanglement without needing a detailed model of the system. This means that their method could be used to control a wide range of quantum systems in practice.
Lai’s research is a step forward in making quantum technologies more practical and reliable. By improving our ability to control entanglement, his work could lead to new advancements in quantum computing, secure communications and other cutting-edge technologies.
We spoke with Professor Lai to learn more about his innovative research and how it could shape the future of quantum technology. Here’s what he had to say about his work, its challenges, and the exciting possibilities it holds for the field of quantum information science.
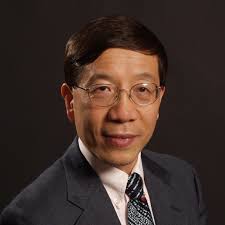
Question: What inspired you to explore this particular topic?
Answer: Exploiting machine learning for controlling quantum systems, especially quantum information systems, is rapidly becoming a promising field of research and is attracting increasing attention.
Q: Can you share any exciting or unexpected findings or breakthroughs from your research so far?
A: Using modern machine learning-based control to achieve any desired level of entanglement between the optical and mechanical modes in optomechanical systems in the nonlinear operation regime is a breakthrough. A highlight is that the developed machine-learning-based control is model-free, where policies or value functions are directly learned from the interactions with the quantum environment without any explicit model of this environment, rendering experimentally or practically implementable the control scheme.
Q: In a broad sense, what are some of the practical implications or potential societal benefits of your research?
A: Entanglement is fundamental to quantum information science and technology, yet controlling and manipulating entanglement, the so-called entanglement engineering, for arbitrary quantum systems remains a formidable challenge. The ASU work develops a model-free, data-driven, and deep reinforcement-learning-based feedback control framework to create and stabilize entanglement from partial observation in quantum optomechanical systems. It is a small step forward in exploiting machine learning for quantum control.
Q: Can you describe the approaches you’re using in your research, and why you’ve chosen these approaches?
A: Quantum control is essential to quantum engineering and technology. Traditional control methods rely on a mathematical model of the quantum dynamics that may not always be available in applications. Modern machine learning provides the possibility of developing quantum control schemes without the need of any model.
Q: Are you collaborating with other researchers or institutions for this project, and if so, how does this collaboration enhance your research?
A: This is a collaborative work with Prof. Christian Arenz from ASU Electrical Engineering and Prof. Joseph M. Lukens from ASU’s Research Technology Office and Quantum Collaborative and Oak Ridge National Laboratory. Professor Arenz is a top expert in quantum control and Prof. Lukens is a world leader in quantum networking. Both collaborators provided tremendous insights and extremely helpful suggestions and comments throughout the research.
Q: What advice would you give to an undergrad or grad student interested in pursuing research in quantum information science?
A: Developing ways to control and manipulate quantum systems using modern machine-learning methods is cutting-edge research that will lead to new technologies to benefit society in a fundamental way. Tremendous opportunities will arise for undergraduate and graduate students in science and engineering. The research is interdisciplinary, requiring knowledge about quantum mechanics, machine learning, and control theory. A solid training in basic physics and mathematics will be essential if a student intends to develop an exciting career in quantum information science and technology.